“Can we come up a policy that, if not fast, at least reach the goal!”
Background
Stochastic Shortest-Path
we are at an initial state, and we have a series of goal states, and we want to reach to the goal states.
We can solve this just by:
- value iteration
- simulate a trajectory and only updating reachable state: RTDP, LRTDP
- MBP
Problem
MDP + Goal States
- \(S\): set of states
- \(A\): actions
- \(P(s’|s,a)\): transition
- \(C\): reward
- \(G\): absorbing goal states
Approach
Combining LRTDP with anytime dynamics
- run GPT (not the transformer, “General Planning Tool”, think LRTDP) exact solver
- use GPT policy for solved states or visited more than a certain threshold
- uses MBP policy for other states
- policy evaluation for convergence
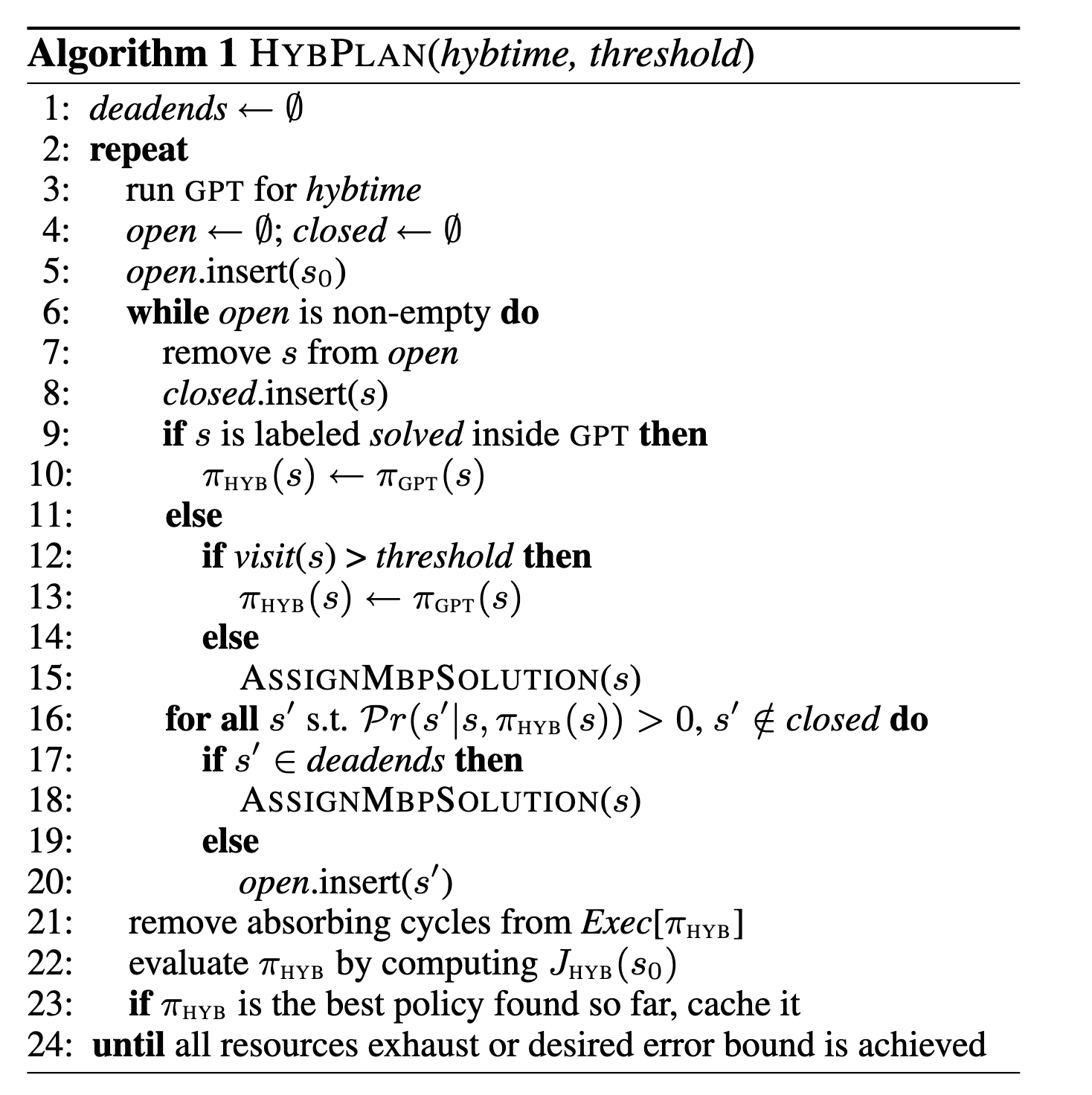
“use GPT solution as much as possible, and when we haven’t ever visited a place due to the search trajectories, we can use MBP to supplement the solution”