DOI: 10.3389/fcomp.2020.624488
One-Liner
Used an ERNIE trained on transcripts for classification; inclusion of pause encoding made results better.
Novelty
- Instead of just looking at actual speech content, look at pauses specific as a feature engineering task
- \(89.6\%\) on the ADReSS Challenge dataset
Notable Methods
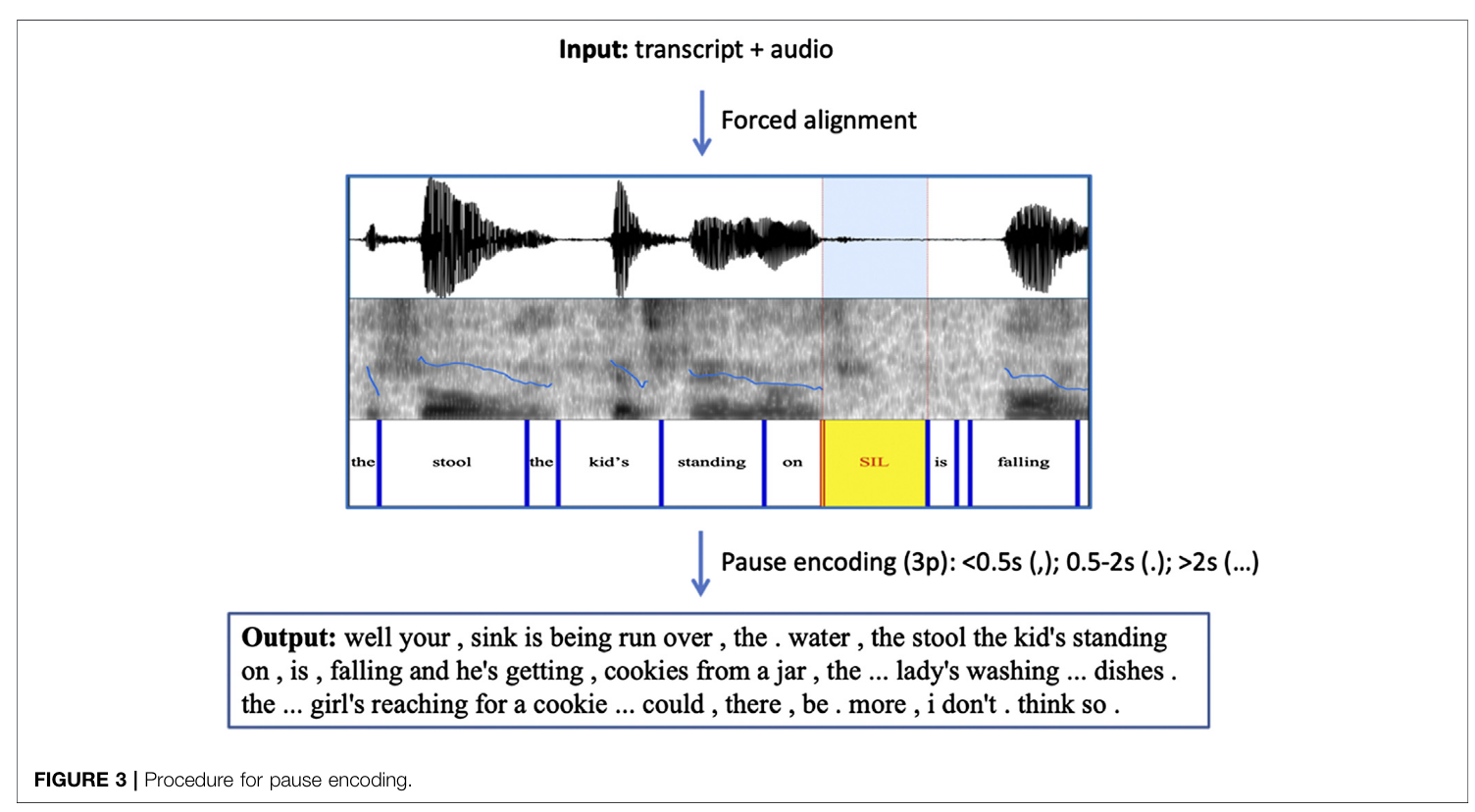
Applied FA with pause encoding with standard .cha
semantics (short pauses, medium pauses, long pauses). Shoved all of this into an ERNIE.
Assay for performance was LOO
Key Figs
Fig 1
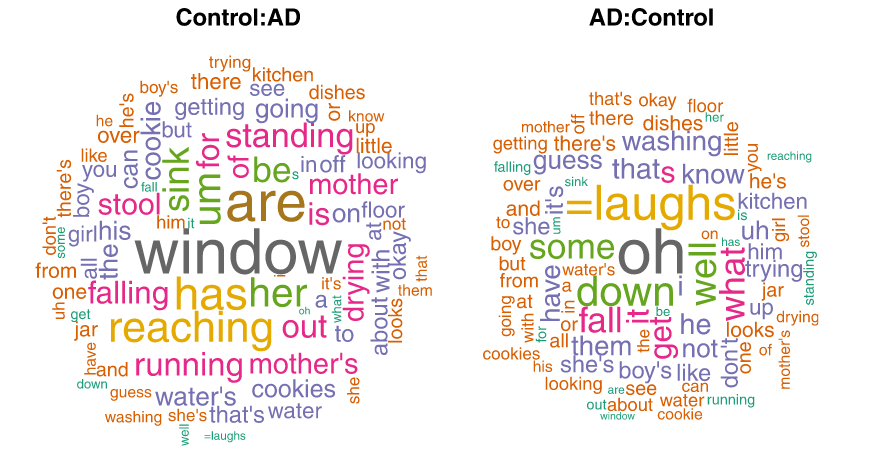
This figure motivates the point that subjects with AD says oh and um more often; which prompted Table 1
Table 1
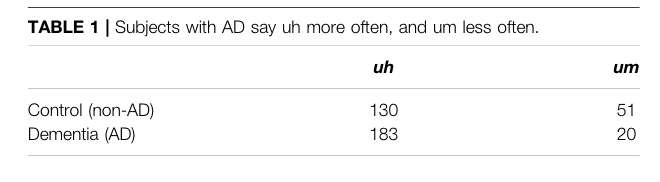
Subjects with AD says uh a lot more often; no significance level calculations but ok.
Figure 5
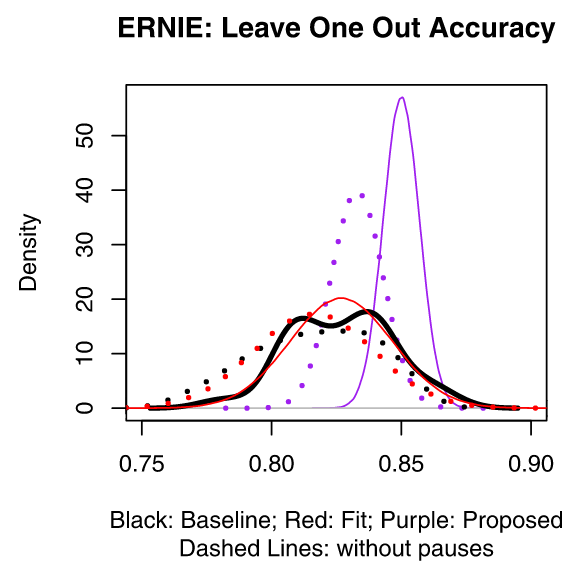
This figure is the result of a LOO study on the proposed model and presumably others before. X axis is the validation accuracy in question, Y is the density by which the score in X appears in an \(N=35\) LOO measurement.
This figure tells us that either way the ERNIE model is better than state of the art; furthermore, transcripts with pause encoding did better and did it better more of the time; that’s where the 89.6% came from.
New Concepts
Notes
Glorious.
